Shift Work Summit featured a session led by Saurabh Das, VP of Engineering at HyperTrack, as he discussed the nuances of no-show detection in shift work. With a focus on healthcare marketplaces, Saurabh was able to break down the complexities of implementing no-show detection at scale, offering valuable insights for businesses grappling with this common challenge. Drawing from his working with the largest shift work marketplaces, Saurabh provided an overview of how industry leaders think about the no-show problem and the solutions they implement to boost reliability and ensure every shift is successful.
Read the transcript below and watch the full video to learn how shift work marketplaces improve visibility by 41%.
Check out the highlights below and watch the replay for details.
The Importance of Location Intelligence
Signals to Achieve True Visibility
The Trifecta of No-show Detection
Reliable Location Tracking is Achievable
How Shift Work Marketplaces Improve Reliability
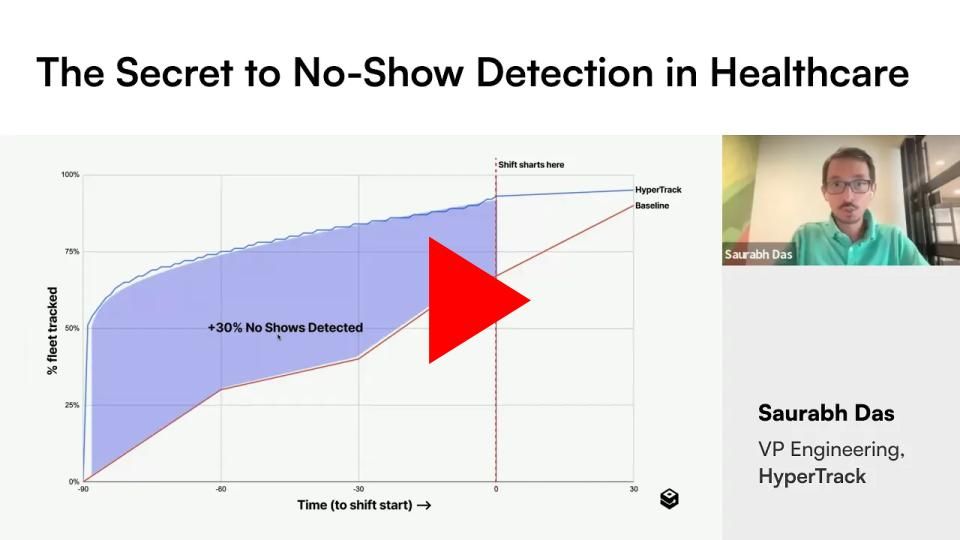
Gaurav Deshpande: All right. Wonderful! Welcome back!
Thank you for staying with us here at the Shift Work Summit.
We are in the last three sessions, and this one is particularly exciting for me; because when I started talking with shift work marketplace companies, while speaking with product managers, engineering managers, ops managers, there are two words that strike fear in the heart of every manager in a shift work marketplace, and those two words are no-show.
When a worker signs up for a shift and does not show up, does not call, this is an egg on the face for the shift work marketplace. They lose the revenue, and can also lose the customer.
So it's one of the most important problems we address. Shift work marketplaces have brought amazing freedom and flexibility in the hands of 50 million workers across all industries.
They can choose where they work when they work, and how much they get paid. But with great power comes great responsibility.
When you have hundreds of thousands of workers going from their homes to thousands of customer locations, the platform must rely on automation to validate the workers, showing up on time, and then spend the time that they are paid for to make sure that they actually spend the time that they're paid for on site, doing the work.
The impact of a no-show or a shift abandonment is especially acute in healthcare, where this involves human lives and well-being.
Join me as I welcome HyperTrack’s VP of Engineering, Saurabh Das, Saurabh helps our largest marketplaces validate shifts at scale. In this talk Saurabh will give you a peek under the hood, and share insights on what he has learned from managing millions, over 3 million, shifts per year for healthcare, and other industries.
So, over to you, Saurabh, the stage is all yours.
Saurabh Das:
Thanks, Gaurav. Hi, everyone. I hope you're having a good Shift Work Summit so far. Yeah, we're going to talk about. You know the secret to not show detection.
And – spoiler, it's essentially location reliability.
And while you know this. the lessons here are generally applicable.
We're talking about healthcare. And because all the numbers and statistics here are taken from our few customers in this sector. So in that way it's specific.
So I mean, you already know the secret. But well, let's go through it, anyway.
So you know, shift work marketplaces in general, you know whether it's healthcare or not, you work with a guarantee that you're going to get the service that you know you're signing up for.
The person is gonna show up and stay there for however long the shift is.
And shift workers are becoming an essential part of the workforce in many companies. And therefore it's critical for these businesses to have reliability in in order to plan and execute their businesses correctly.
The importance of location intelligence
So, what I'm basically saying is that it's really important to make sure that the person shows up and that they stay there.
So, what I'm basically saying is that it's really important to make sure that the person shows up and that they stay there.
At the same time, you know, some stats are somewhat shocking. Which is that what almost half of all us employees admit to time theft, theft, and it's like a 11 billion dollars problem.
And that doesn't even include mistakes. So this is, you know this, it's even higher than that.
But at the same time there's a real pressure. You know, if you have to guarantee the quality of workers there, you know you have to go through an expensive process to make sure that they they fit a quality bar. So you know quality of workers, miscommunications and bad actors all essentially, really stress the system for shift workers more than you know, regular full time employees. and therefore it's even more important to make sure that you know the surprise.
A no-show is the surprise.
As well as shift abandonment issues.
Those must be reduced and tackled in some way and location intelligence is one of the ways you can tackle it. It is one of the key ways in which a lot of market marketplaces are tackling the problem of you know when when it shows up.
So let's have a look at you know what you do in a case of that, or what you'd like to do to add location intelligence to your marketplace.
And as we said, pre-shift before the shift starts, the main risk is no-shows. So you want to kind of track that location. If they're not, gonna show up, you backfill it. Similarly, when once they arrive, they're working.
How to Add Location Intelligence to your Infrastructure
You track their location constantly, and if they leave the shift without an alert. you have, you have the option of backfilling alert the customer, etc. And you know that that sounds like that sounds reasonable. So you want to do that.
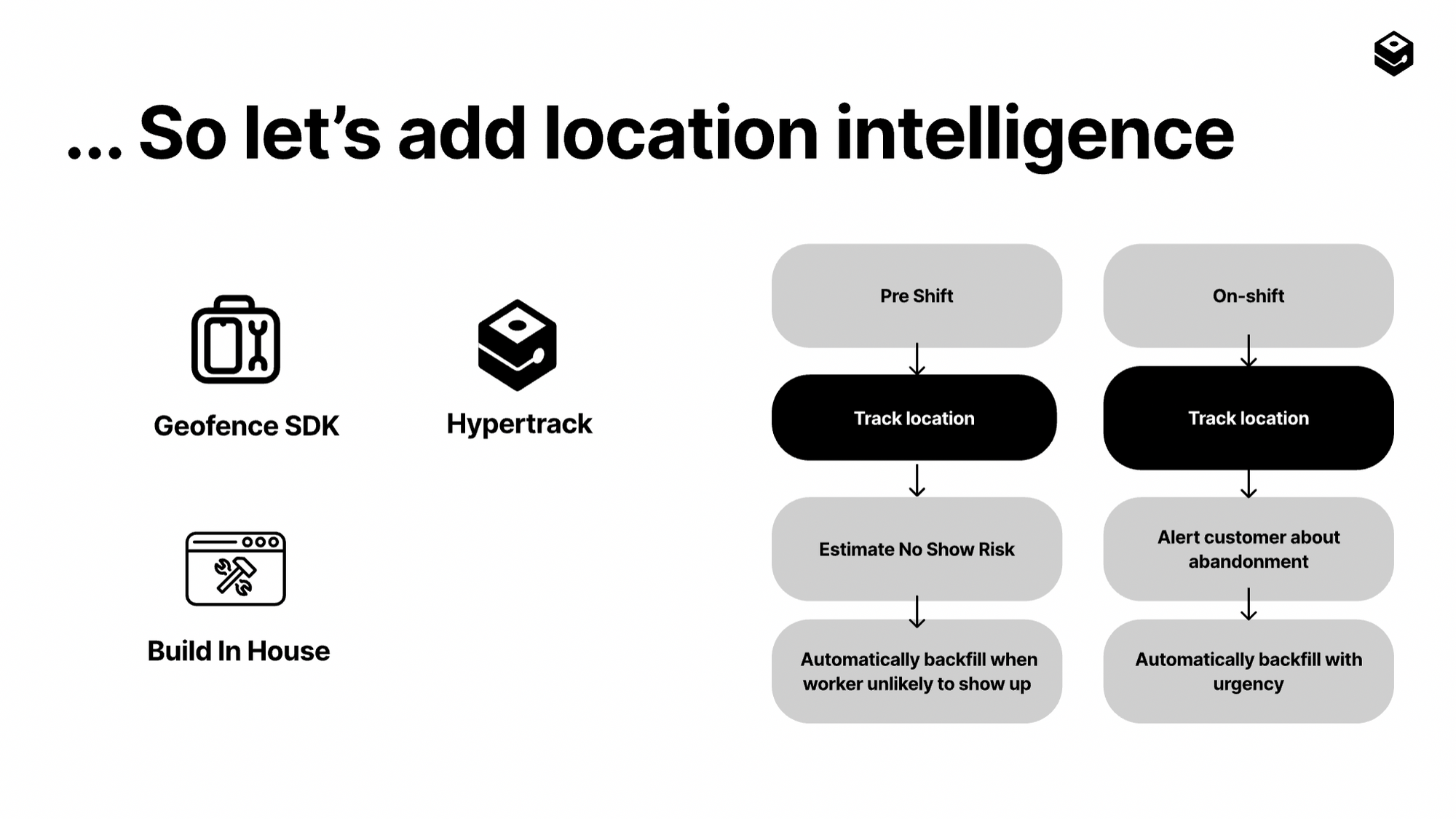
Now, there, there are quite a few options to add location intelligence into your app right now.
So you have geofence SDKs. You could also think about building it in house, and of course there's HyperTrack.
All of them have, you know, target some use cases, some of, and have some advantages and disadvantages.
And, it sounds pretty easy, right? You know we get this done, we'll add this and we'll have our locations. But there are, of course, a bunch of other things to think about that impact whether you actually get the location.
You know things like you need to wake the devices up remotely. So that is there in both your in-house solutions as well as if you use geofence. SDK, kind of solution.
You also need to be able to minimize the battery consumption.
Otherwise, your workers aren't going to agree to track issues. You also don't want to track it when they don't expect to be tracked. They lose faith in the app, and they stop giving the permissions.
You also need to be able to detect permission changes for various reasons, but also for fraud cases where people are likely to change the permissions before they do something that you don't want them to do.
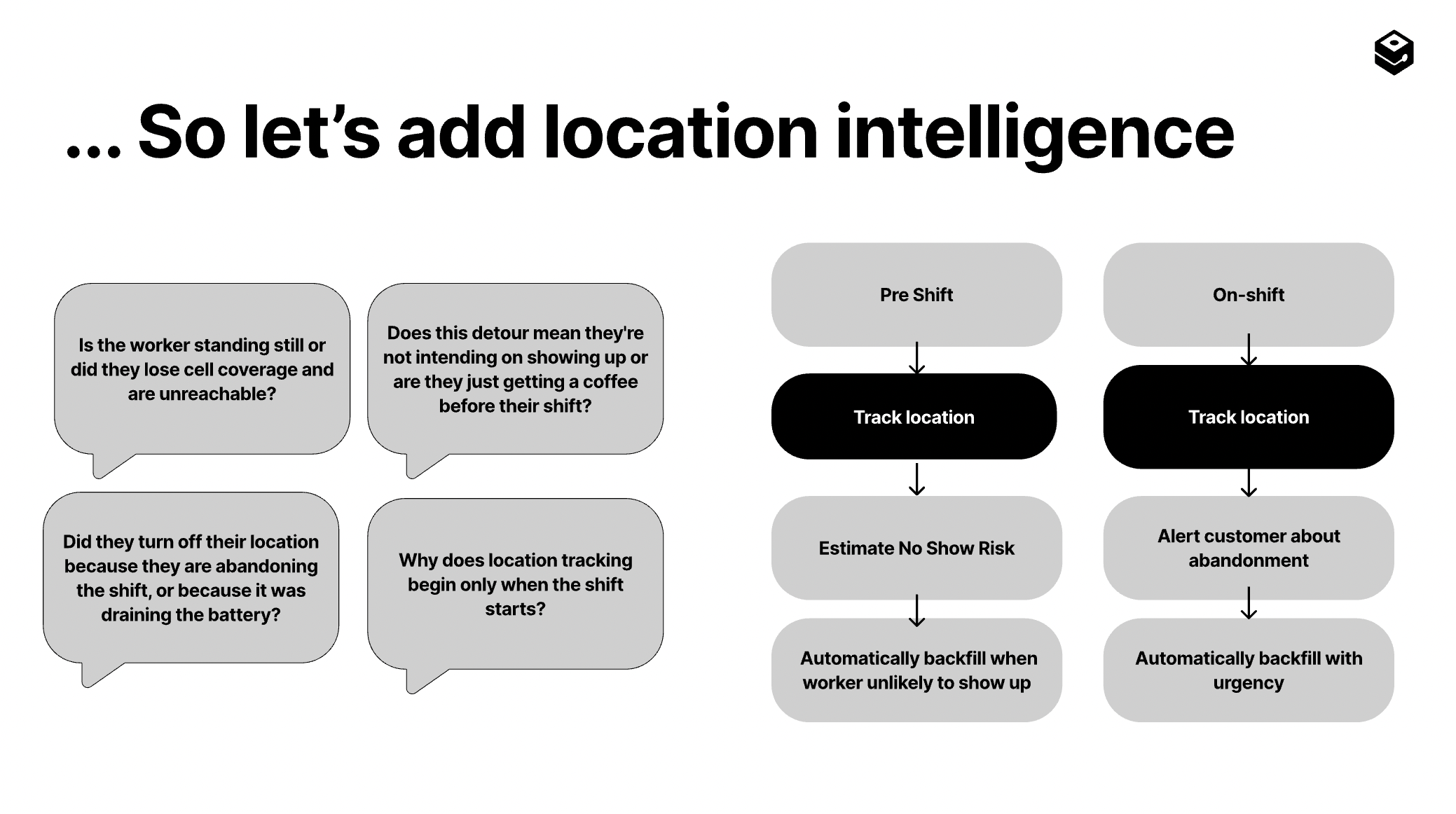
There's also adversarial issues, mock locations, and all kinds of things there.
But the biggest chunk of work falls into this category of you know, we have two main OS's and one of them has thousands of different kinds of devices, and each one has its own quirks. It's, you know, you have to a little bit of work here and there to make sure that sort of each of them is tracking correctly.
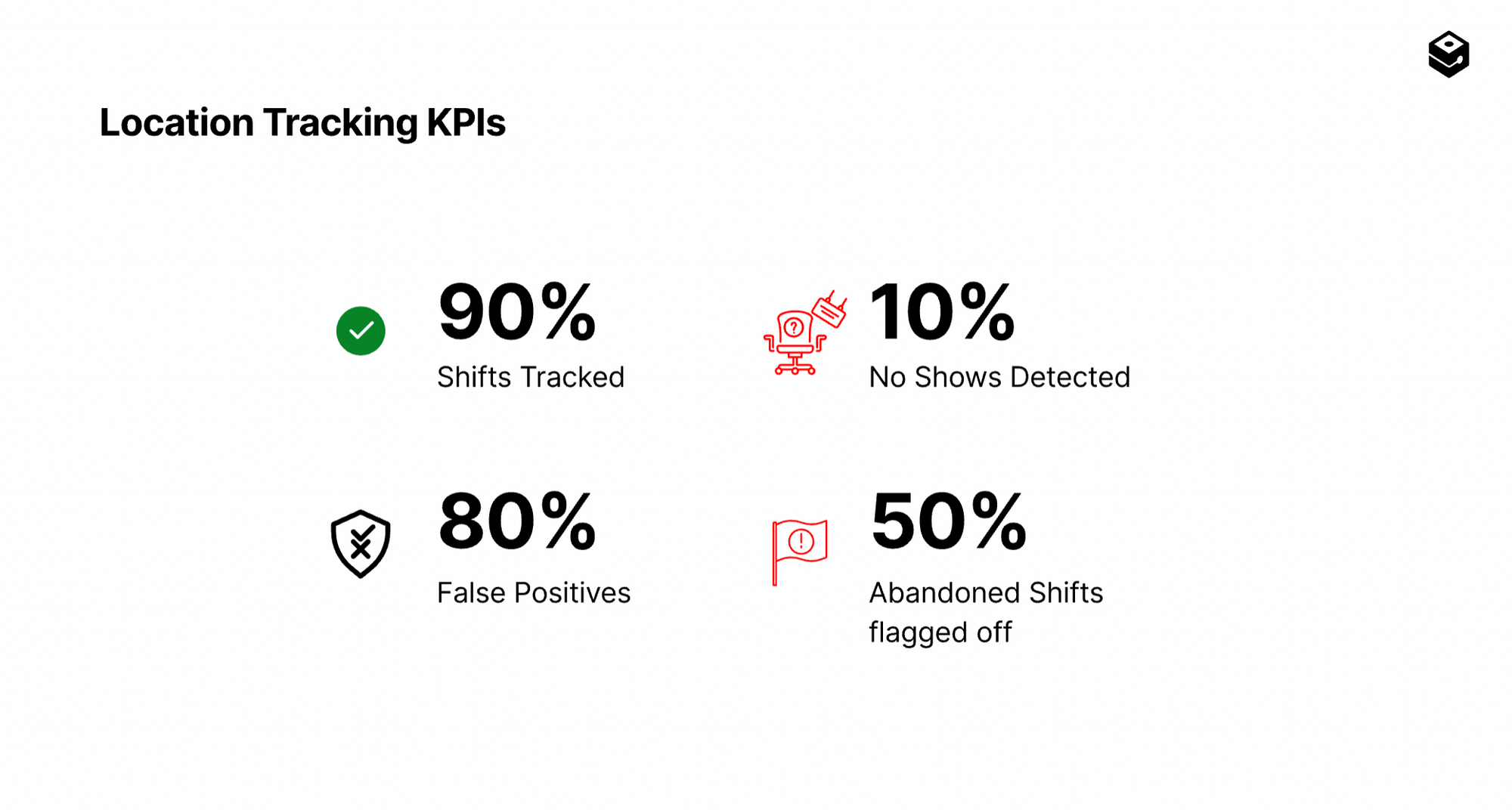
So that's that's the other thing that you have to deal with. So you know there's a good amount of work that goes into it. But you know you could do that, and you start tracking.
But then there's more nuanced questions around the intelligence portion of it,the mobile devices and moving, so are they actually standing still?
Or did they lose coverage, or did they turn off their permissions?
You know what's going on there, and they're at the edge of the facility, so should I mark them as a no-show. Should I think that they're still gonna come like, what do you do?
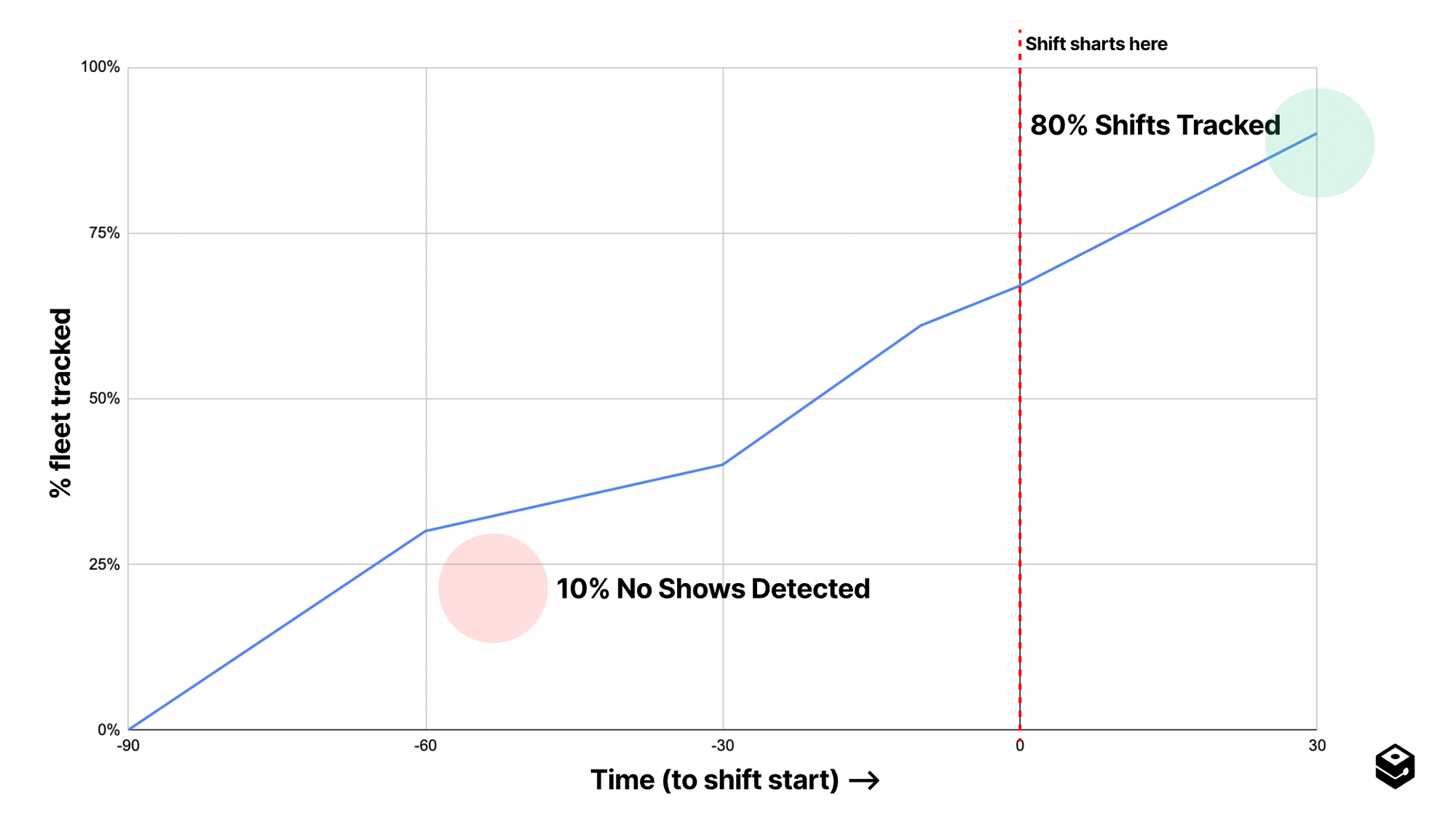
The other one, which we see very frequently is, you know, you're watching on the map, and they take a detour. And does this detour mean that they're not gonna show up? Or does it mean that they're getting a coffee before their shift. And it's a hard problem to solve this one.
and there are other things, you know, adversarial issues I mentioned. Like they're are, they turning off their battery, their tracking because their battery is low, or is it because they want to abandon the shift?
And they've therefore they're gonna track it off and then leave and the other one is that you know you're trying to track your shifts and get to no-show detection.
But you start getting locations only when the worker arrives at the shift. So what's going on there?
So all these kinds of questions need to be dealt with, and you know, say, you do that. And you start tracking your workers. And you get to a place where you say that 90% of my shifts are being tracked for some definition of being tracked is the key.
Because you see that even though 90% of your shifts are tracked, you get only 10% of in your shows being detected correctly at the same time, you have 40. You know, you have 80% false positive in your no-shows.
And you also notice that even though 90% of your shifts are tracked, only 50% of abandoned shifts are being detected. So you know, despite having sort of good tracking and spending a lot of effort doing that.
You don't get the business outcomes that you want here, and the brute force implementation with geofence SDK that gives you locations, it typically gives you something like this.
And because waking up devices reliably is a hard problem.
So yeah, I mean, how do you explain this?
And you explain this by thinking about your metric?
You're looking at an 80% shift track. But that could happen even after the shift starts. What you need to do is look at what happened before the shift starts, and when, how how long before the shift started.
It happened so the blue line you see here is a line that shows you how your devices in your fleet woke up, typically as you started attempting to track them.
So, as you can see, you know, after shift starts, you eventually reach a number which looks very rosy. You see, 80% of your shifts attract.
But it took a really long time to get there. You know, you track them for 90 minutes, and when the shift started. So after 90 minutes are over, you're still only tracking something like 65-70% of your shifts.
But you actually want to detect your no-show somewhere here around 1 hour before your shift starts, so that you can actually backfill on time.
So this region kind of gives you 10% of your no-shows. And that's kind of what we're seeing
in the previous metric. So where we want to get to is.you know, HyperTrack has spent a lot of effort trying to wake up devices. Waking up devices is a hard problem.
But you know, with all the work that we've done. We’ve we. We get a curve like this is pretty typical for typical for many of our customers.
And you know, around an hour before you kind of wake up 75% of your devices gives you a good chance of detecting a no-show.
And what you do see is that indeed? You get about 30% more. no-shows detected.
You know, at in in this in this region. So you cannot get to around 40% directly here. And on top of this there needs to be more work to get it to 60-70%.
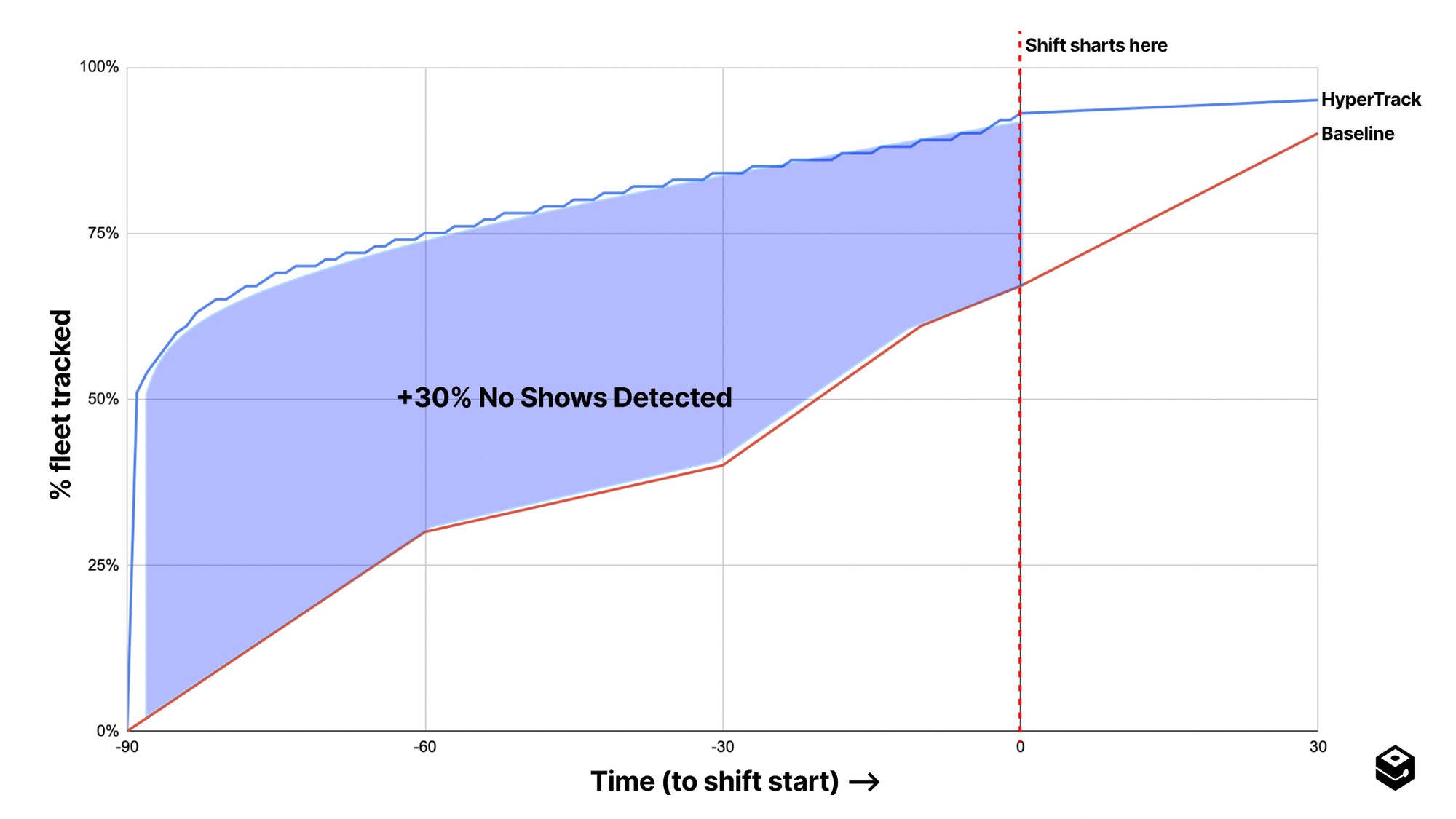
But even, you know, if you look at this this march there is a little bit extra that you can get directly from HyperTrack, and the key here is that there are times when you can reach a device.
And you still can't get a location - out here the blue line gives you when you were able to get a location for the first time from the device.
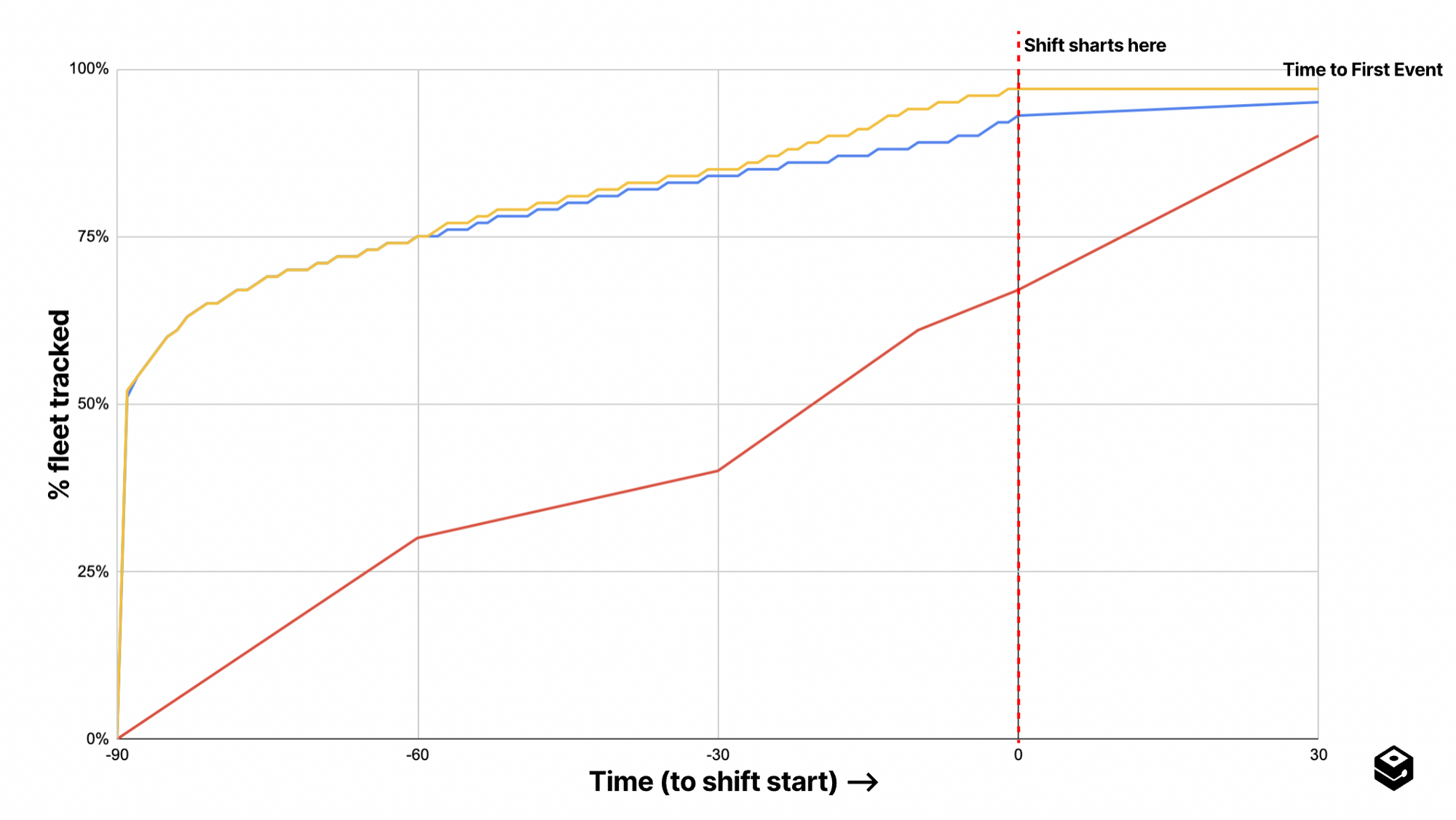
But the yellow line, which is almost coincidental, tells you that there are some devices which you could reach, but you couldn't get a location from them, most often because the location permissions were denied.
Signals to Achieve True Visibility
“When you think about shift abandonment where people are often likely to switch their devices off, or stop giving permissions just before they abandon a shift. So having these signals, even if you don't have the location is a very important piece of knowing that.”
And you know they seem pretty pretty close. There's like hardly a percent or 2 between them. So you know, we probably have to go on a zoom in to see if there's any difference. But what we do know is that, 10% of your no-shows come from this one to 2% additional piece of information. So yeah, I mean it. It's a small number in terms of your overall shifts, but it's a very large number when it comes to the proportion no-shows.
So what we're saying is fleet visibility, you know, even without location, is an important piece of the puzzle.
And you know, HyperTrack in his mission to, you know, serve the shift work marketplace, essentially breaks down reasons for not being able to reach your devices into various categories.
And you know, for the first three we have the ones in green. Your ops. managers can get in touch with the users and get them to change settings, or tell them not to close the app or whatever else, and get them to resolve these. So these are resolvable.
And the other two, the reachability stuff can be resolved by talking to your developers, you know, typically around push notifications and other features.
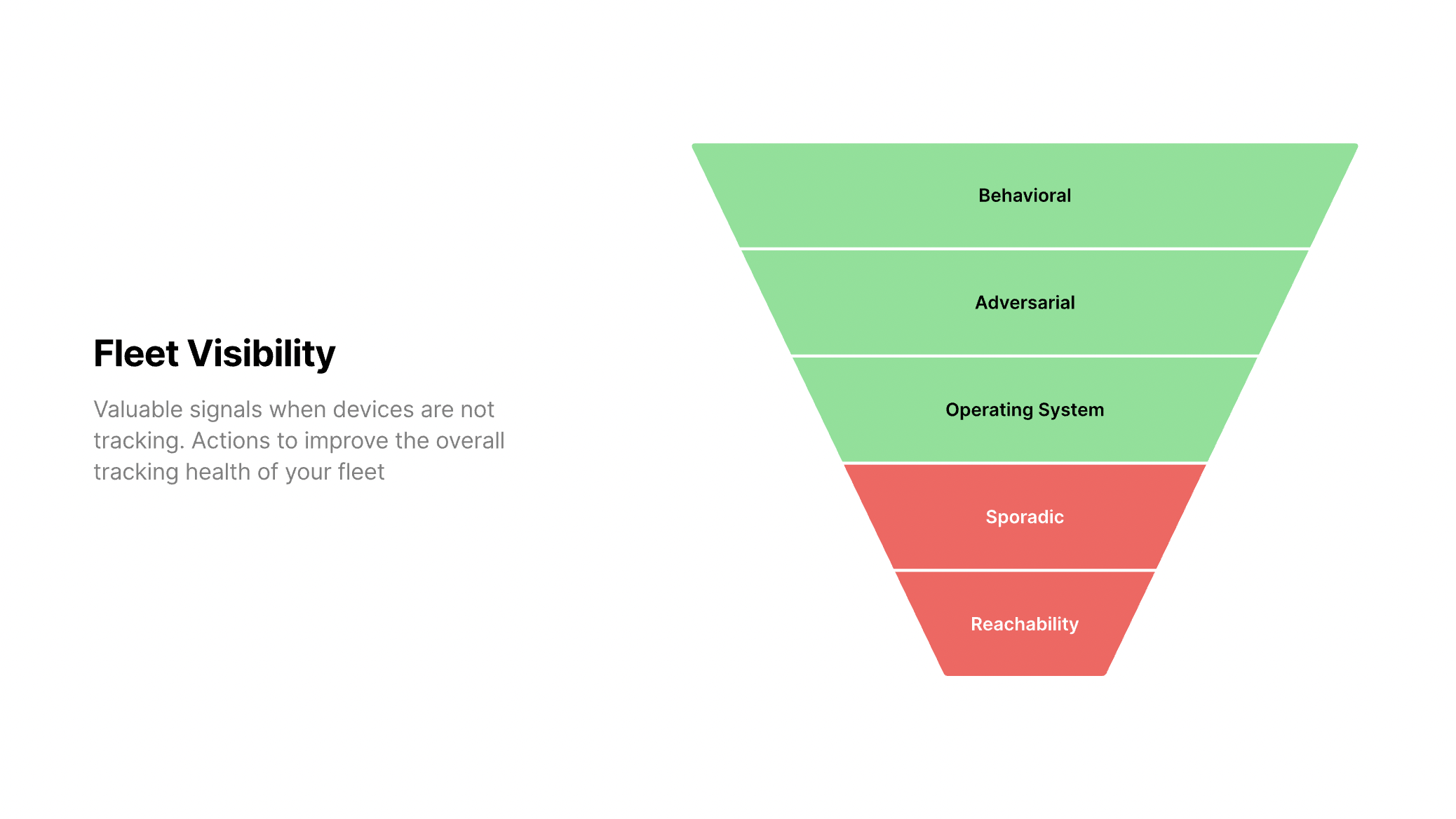
So I think the key here is that this bit gives you a good 10% additional, no-shows. And it's even more stark when you think about shift abandonment where you know, people are often likely to switch their sort of devices off, or stop giving permissions just before they abandon a shift. So having these signals, even if you don't have the location is a very important piece of knowing that.
The Trifecta of no-show Detection
“The trifecta of, improving no-show detection is essentially focus on the time to first event, actionable outages, and tracking consistency.”
Yeah. And you know, a part of this also is knowing whether the device disconnected and turned off? Or is it just unreachable right now? So you know, hyper track tries very hard to also give you clarity about that piece of it.
So you know you put all these together, and you can get to you know, a good amount of your no-show no calls detected pretty easily.
On top of that you have to, you know, get better algorithms get better machine learning stuff going for your specific problem. And you can get to much higher numbers. Once you sort of start tackling it from that angle.
So, with this, you know the what's the the trifecta of, you know, improving. no-show detection is essentially focus on the time to first event. How quickly you can wake up your devices.
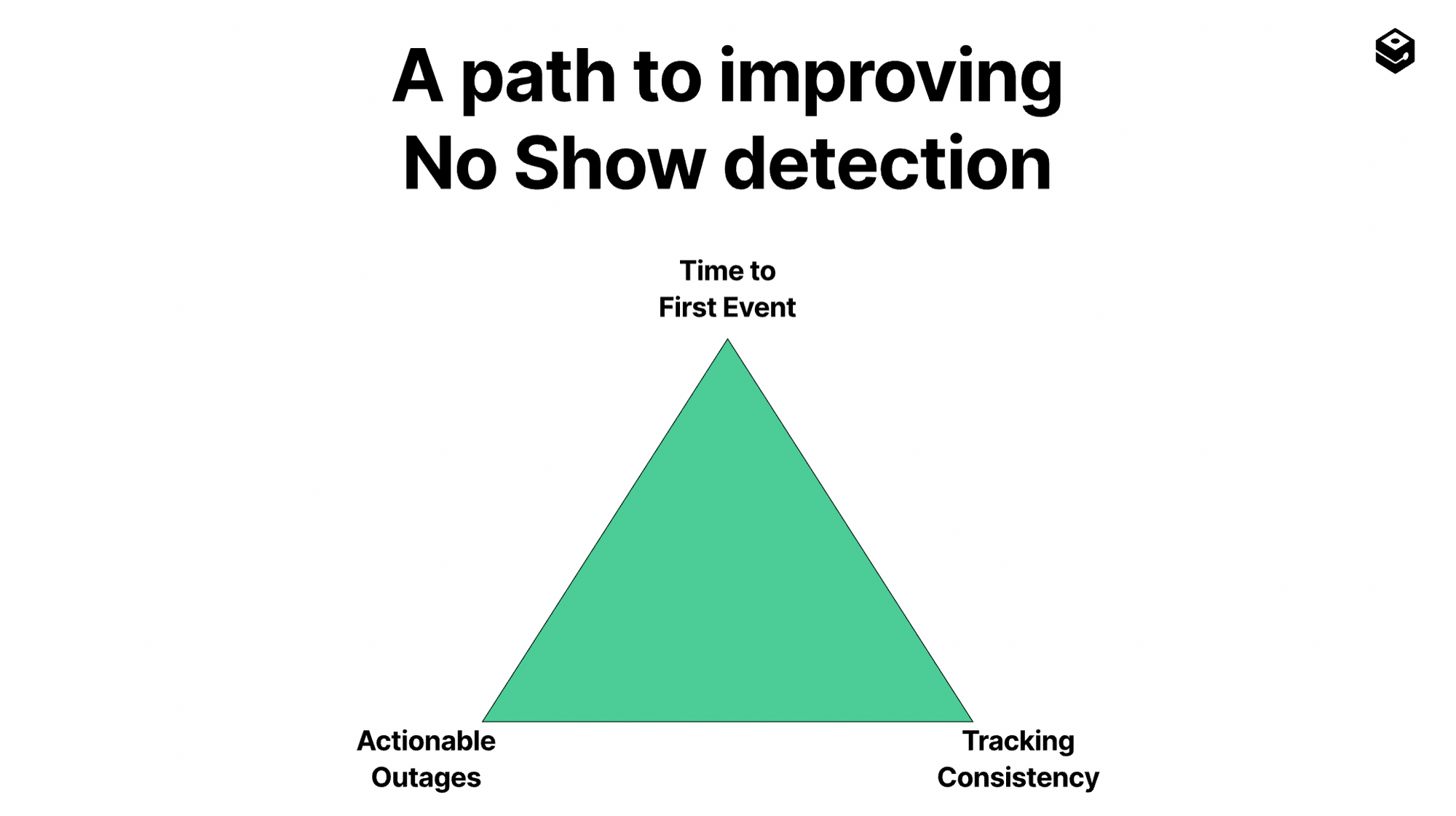
Actionable outages – so information about why your devices aren't tracking right now; and tracking consistency, which is once you start tracking, do you continue tracking? So this is a problem often faced with in house solutions, where you're able to get it to start tracking reasonably well. But then it gets turned off for various reasons.
So you have to work hard at also ensuring that it stays tracking, and the OS. Doesn't throttle your app, etcetera. So that's the other part of it. and actionable outages also has another important part, which is that it gives you recommendations about what you can do to you know improve the health of your fleet, so you can tell your users to.
Reliable Location Tracking is Achievable
“It's a considerable amount of work, but it's valuable, and you know you have to work both on the location piece of it as as well as the algorithms, and ML to detect the no-shows that are relevant for you.”
So if you know that your users are closing the app, you kind of have to tell them not to do so or change your flow so that it's not.
So they don't close it as often. Or, on the other hand, if you notice that push notifications are an issue.
Then you have to tell your developers to, you know, change the flow there.
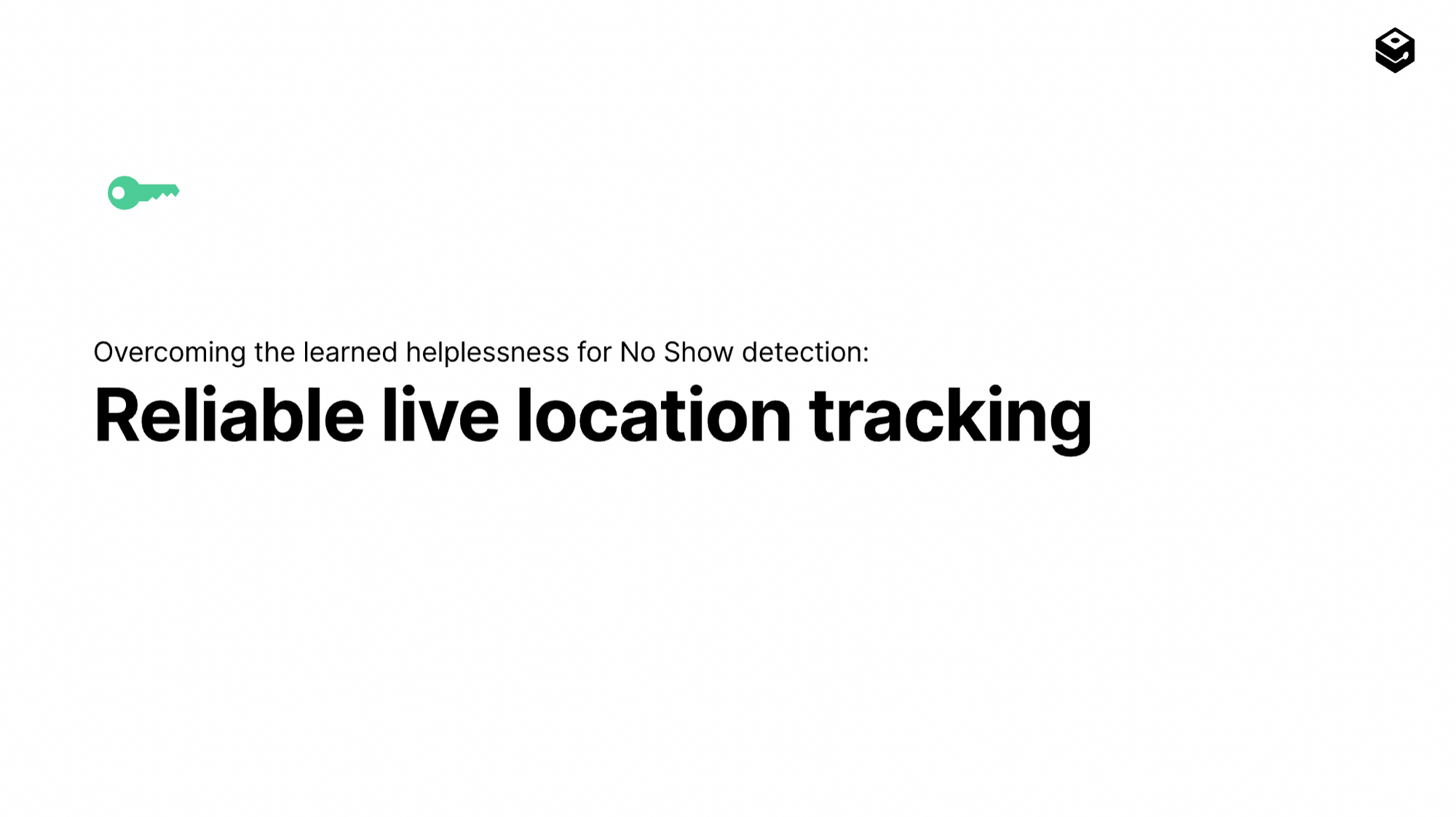
So we categorize our outages and give recommendations on what you can do to sort of improve the tracking health of your fleet at an overall level, and that helps push the. You know theoretical limit of how much No call, no-show, you can detect higher and higher.
So you know, we haven't discussed tracking consistency much here. But I guess, you can take a stab at that. It looks like you know, things like OS is killing the app because it's taking too many resources or incorrect permissions, or, you know, too much resource, consumption, etc, and HyperTrack looks at some of these issues and provides guidance.
But also in some cases where you know, you're unable to solve it. Our mobile team is able to provide insight into why, your app is crashing, for example. You know, when they have nothing to do with the HyperTrack SDK.
How Shift Work Marketplaces Improve Reliability
"TL:DR we're seeing much better "location data reliability" with HyperTrack, seeing device reachability improvements of 41% an hour before shift starts”
So it's, I said, a path to improving no-show detection. But it's a considerable amount of work, but it's pretty valuable, and you know you have to work both on the location piece of it as well as sort of algorithms, and ML to detect the notions that are relevant for you.
You know it's hard work, but it is very doable. We've seen a lot of customers who’ve been very successful with this whole effort.
And I'm gonna sort of leave you with some of the words of our customers.
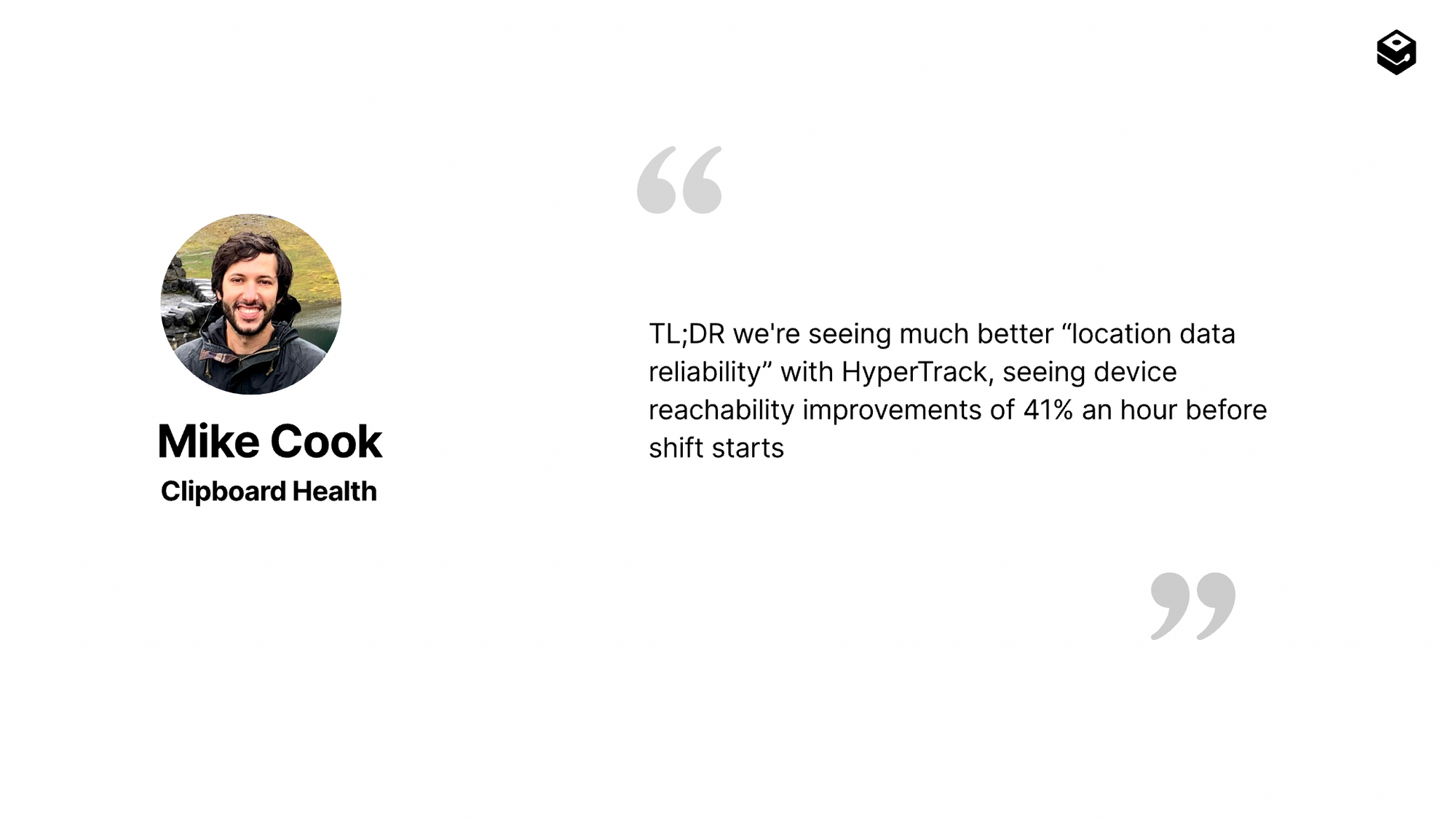
To quote Mike Cook, who's really pushed us to improve the reliability and robustness of our platform, and he's ensured that we've left our platform in a very good place now.
You know, he he did say, that we're seeing significantly improved location data reliability with HyperTrack and it was great to work with the team here to get to get to this stage.
Also, I want to really thank Jason, who’s helped me think through this presentation, and also about the whole problem, and how we can address and modify our platform to work really well for healthcare marketplaces.
And you know, Jason, sees Clipboard as a leader in the technology portion of marketplaces in the shift work movement, and hopefully he'll be here next year, presenting at the Shift Work Summit himself.
So, that's the key to it, you know. How do you? There's a certain amount of learned, helpless helplessness around no-show detection. A lot of people have told us that.
And you know the key to overcoming it is just reliability, reliable live location tracking.
A lot of effort has to go into it, but it does yield results at the end.
And you know, I'm sure you guys have a lot of questions. So with that throwing the floor open.
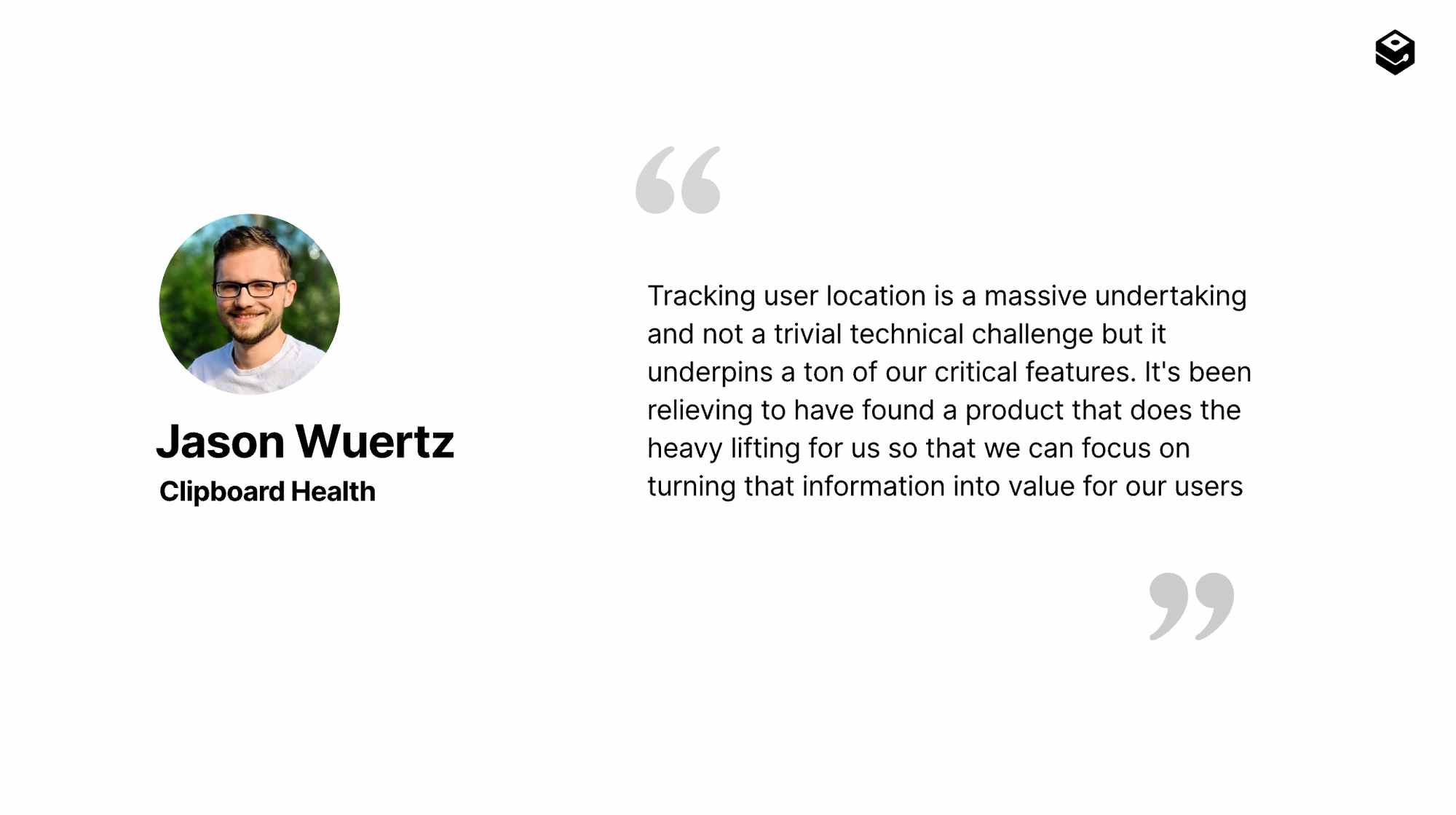
Q&A led by HyperTrack CMO, Gaurav Deshpande
Gaurav Deshpande:
Yeah, yeah, there are quite a few questions, sort of first of all, thanks a lot for taking the time to walk us through this. It's wonderful to see.
Wonderful to see engineers’ testimonials from customers we work with multiple customers. Clipboard has been awesome.
Nursa, one of one of the other customers in healthcare we work with presented earlier as a part of the Shift Work Summit.
One question related to the tracking you mentioned that tracking rate improves dramatically with HyperTrack. Can you talk about? Why, many of the shift work marketplaces here are using location tracking without very good results. Can you talk about What's the difference between those 1st generation location tracking technologies and what hyper track provides?
Saurabh Das:
Yeah. So I think, there is a specific need to understand the metrics that you want to track and you know, and sort of separate out the tracking rate metrics from the business impact metrics.
And by separate out. What I mean is that the tracking rate metrics, without addressing the business of metrics is not very helpful.
So with each customer there are myriad of problems in in the tracking. You know, space and things can go wrong in hundreds of ways, right?
And each of these subproblems needs effort to solve and for different customers a different subset of problems is relevant.
Solving all of them yourself is very hard and that's where hypertrack has as a leg up, because we see the problems of a lot of different parties. And we've spent the effort to handle those edge cases.
So it's it's all about the effort you want to put in right? It's hyper track is not in a way, you know, it's not got magic technology under the hood. That is different from what you call 1st generation tracking, doing it in-house.
It's in the same kind of techniques. It's just that there are so many places you can get stuck.
And it's hard to navigate those spaces and hypertrack just by, you know. Having done this for this amount of time, and having a sort of overarching view, is able to get to all these corners and resolve all of them one by one.
And you know it's it's the hard work that that needs to be put in, and that's a difference. Here.
Gaurav Deshpande:
Excellent. Thank you, Saurabh. Second, next question is about the time to first event you mentioned that term.
There was a clarification question about that from the audience. Can you classify that a little bit further? And how that relates to the no-shows, and how that factors into the overall flow.
Saurabh Das:
Absolutely let me let me go back here so time to 1st event, essentially so, there's time to 1st location and time to 1st event.
So look, what are we trying to do, or where do we start? We started at? Oh, if you know the location of the worker, we can detect no tourist.
and you did that, and you know you got the blue line here, which is that you start? Try, try to wake your entire, you know if where you you have a shift at 10 o'clock.
One and a half hours before that, you try to wake up the device. You do that, and you know you get this kind of a curve which says that this is the statistical property of my fleet. It's kind of waking up in this manner now. So that's time to first location.
What we realize is that there are often times when we're able to wake up the device so we can reach the device. But the device is unwilling to give us a location.
It is either in an outage state or it's in a constantly disconnected state, or a whole bunch of other issues. There are many reasons why you could read the device at times, but you can't get a location from them.
So time to first event, essentially, is that state where you know you've reached the device at least once. You know that you've been able to successfully ping the device.
But you can't get information from the device. So what's going wrong here?
And it turns out that we are able to get information about why we're not getting information, even if in many cases.
So those that piece of information about, you know why you're not able to get location data is often very telling about risk.
And by using that sensibly, we can, you know that has a. So it's it's a very small number of devices. If you think about it, it's just always like one or 2% of your fleet that would have, you know, time to 1st event, but not a location.
But those few percent of your fleet are sort of very crucial in terms of a very high percentage of risks. So you know, those tend to be workers who have a high-risk profile.
Did I get the question, or was there like something slightly different in there that I could clarify.
Gaurav Deshpande:
No, that's perfect. No, that's perfect. Thank you, Saurabh. That was wonderful.
And there are a lot of shift work summit marketplaces here as a part of the community.
Another part of the audience, if you're looking to look at location, intelligence, and manage no-shows effectively with hyper track, feel free to reach out to us. You can just write to us at marketing@hypertrack.com.
---
For more about Shift Work Summit, watch the replay!